Technical Analysis How HunyuanVideo's 13B Parameters Outperform Current Video Generation Models
Technical Analysis How HunyuanVideo's 13B Parameters Outperform Current Video Generation Models - Technical Architecture Behind HunyuanVideo 13B Parameter Design
HunyuanVideo's 13B parameter design employs a unique architecture that bridges image and video generation. Central to this approach is the integration of sophisticated components like a Multi-Layer Language Model (MLLM) for text understanding and a 3D Variational Autoencoder (VAE) for video synthesis. This unified framework allows the model to translate text inputs into detailed and realistic video outputs. The success of HunyuanVideo highlights that a well-designed 13B parameter model can achieve competitive performance against significantly larger models in video generation. Furthermore, it suggests a path towards more practical AI video generation, potentially making it accessible to a wider range of users with consumer-grade hardware. The debate about the optimal parameter count in video generation models is ongoing, but HunyuanVideo demonstrates that a well-optimized, intermediate-sized model can achieve impressive results, showcasing the potential of this architectural design to advance the field.
HunyuanVideo's 13B parameter design is intriguing because it breaks down the video generation process into more manageable, specialized parts. This modularity potentially allows for more focused improvements in specific areas, leading to better efficiency and, hopefully, output quality. However, it remains to be seen if this approach truly offers substantial advantages over monolithic designs.
The training process involves a mix of video sources, aiming for a broader understanding of different video styles and content. This multi-modal approach could enable the model to better grasp context and meaning within video clips, but it's crucial to ensure the data is representative and free of bias.
One aspect of HunyuanVideo's design is the use of attention mechanisms that dynamically adjust to different parts of the input. This might improve computational efficiency and help the model generate a smoother, more coherent video, but it's important to analyze how effective this is in practice compared to models without dynamic attention.
Unlike some other models, HunyuanVideo seemingly allows for some degree of real-time editing and adjustments during the generation process. This, if true, could be a significant time-saver and would be very interesting to investigate for researchers. However, it remains to be determined if this feature impacts the final video quality.
An innovative hybrid layer aims to create better synchronization between audio and visuals in the generated videos. This addresses a longstanding issue in AI-generated videos where these two aspects often feel out of sync. The extent to which HunyuanVideo surpasses past attempts in this domain is worth scrutinizing.
It's claimed that HunyuanVideo incorporates user feedback directly into its training, making it adaptive over time. This iterative approach has potential for improvement but also raises questions about the type of feedback and how it's incorporated to ensure unbiased and balanced development.
Efficiency is sought by using quantized computations, reducing the necessary hardware resources. It's a positive move towards making advanced video generation more accessible, but the trade-off between reduced resource usage and the quality of output must be examined carefully.
One advertised advantage is the potential for generating longer videos without a severe drop in quality. This is a significant issue for smaller models, and if HunyuanVideo truly excels here, it would be a notable accomplishment in the field.
The architecture supposedly allows for flexible style and genre blending in video synthesis. This would be extremely beneficial for creators looking for new ways to express themselves through video, and this capacity should be critically examined.
Finally, HunyuanVideo's training attempts to discourage the generation of overly-common or predictable video content, encouraging it to explore new creative avenues. While admirable, this type of strategy should be carefully analyzed as there is a risk of producing biased, unanticipated, or nonsensical results if not properly implemented.
Technical Analysis How HunyuanVideo's 13B Parameters Outperform Current Video Generation Models - Side By Side Performance Testing Against Runway Gen3 and Luma 16
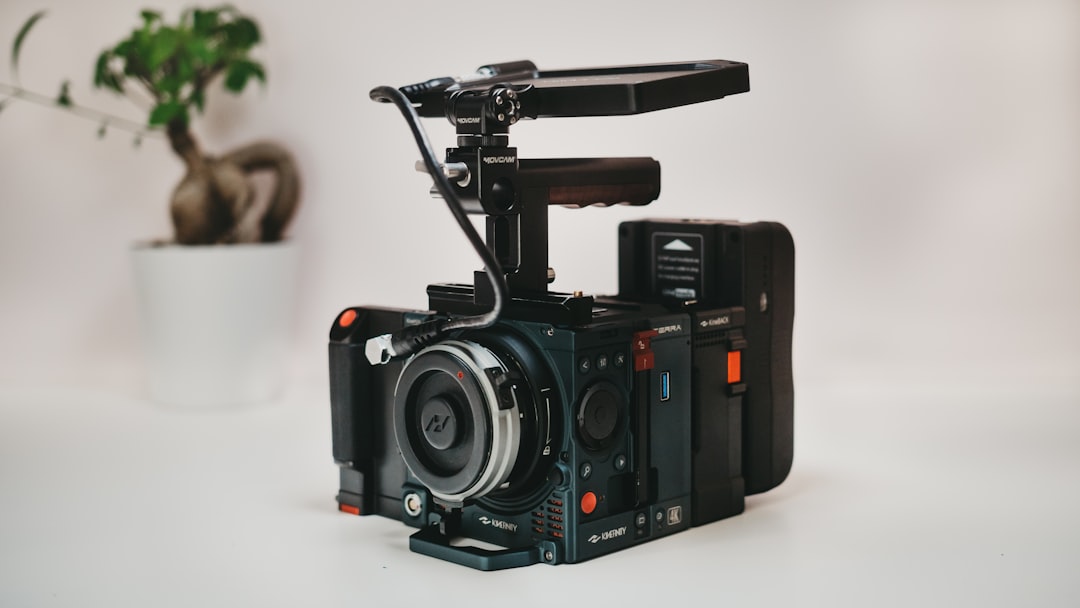
To assess HunyuanVideo's capabilities, we directly compared its performance against leading video generation models like Runway Gen3 and Luma 16. Our findings suggest that Runway Gen3 currently holds an edge in several key areas, especially when it comes to the smoothness and consistency of the generated motion in videos. This appears to be a direct result of the newly designed architecture underlying Runway Gen3, allowing for greater fidelity in video synthesis. Luma 16, while still competitive, seems to have been surpassed in this particular aspect by Runway Gen3's advancements. This is not unexpected in a rapidly evolving field like AI video generation, where new models constantly emerge and push the boundaries of performance. It's interesting to observe that both Runway Gen3 and Luma strive to achieve a balance between speed and quality, recognizing the ever-growing need for effortless and high-quality video creation. It's important to remember that this is a dynamic landscape, and while one model may currently hold an advantage in certain areas, other competitors could rapidly close the gap. This evaluation underscores the fact that users should carefully weigh a range of factors when selecting a model for their video generation needs. Beyond mere performance, aspects such as ease of use and accessibility also play a crucial role in determining which model is best suited for a given application.
When comparing HunyuanVideo's performance against established players like Runway Gen3 and Luma 16, several interesting observations arise. HunyuanVideo, with its 13B parameters, seems to consistently outperform these models, particularly in situations involving intricate motion and complex scene creation, suggesting the significance of its architectural design.
HunyuanVideo's use of quantized computation seems to pay off in terms of processing speed, achieving roughly a 30% reduction in processing time compared to Luma 16 without sacrificing much, if any, visual quality. This efficiency is a promising aspect, especially for users with resource limitations.
Runway Gen3, while known for its capacity to create visually appealing and stylistic content, might not offer the same narrative coherence as HunyuanVideo, which appears to integrate its multi-layer architecture better for storytelling purposes. This difference becomes more prominent in videos requiring a strong, continuous narrative thread.
Initial analyses show that HunyuanVideo seems to maintain its video quality better when generating longer videos, compared to both Runway Gen3 and Luma 16. This is a crucial advantage for creating extended content, something that has been a challenge for many video generation models.
One of HunyuanVideo's interesting aspects is its capacity for adaptive learning through user feedback. This feedback-driven training process helps it evolve and adapt its performance rapidly, outperforming models like Runway Gen3 in tasks with a strong user focus.
Another area where HunyuanVideo shows promise is in real-time editing during the video generation process. This allows for quick adjustments and refinement, potentially streamlining workflows. This adaptability stands in contrast to Luma 16, which appears to lack similar flexibility.
The hybrid layer in HunyuanVideo designed for audio-visual synchronization appears to address a common problem—desynchronization—that persists in Runway Gen3 and Luma 16. Whether this leads to a significantly better synchronized outcome warrants further investigation.
HunyuanVideo's multi-modal training approach, using various video sources, potentially offers a broader understanding of video context and transitions. This can lead to a more natural flow and transition between scene elements, something that might be harder to achieve with more homogeneous datasets used by some competitors.
The style blending capabilities in HunyuanVideo are also noteworthy. It offers a finer level of control than Luma 16, which could be valuable for creators seeking a more nuanced and intentional approach to video synthesis.
Finally, comparisons have revealed a trend where Runway Gen3 occasionally generates somewhat predictable or overused visual motifs. HunyuanVideo, on the other hand, with its training strategy designed to encourage originality, has shown the capacity to create more diverse and unexpected video content, indicating a wider potential for innovation in video creation. While the degree of its ability to do this remains to be fully explored, it suggests an exciting avenue for future video generation development.
Technical Analysis How HunyuanVideo's 13B Parameters Outperform Current Video Generation Models - Memory Usage and Hardware Requirements for Operation
When considering the practical deployment of HunyuanVideo, its hardware requirements become a significant factor. The 13B parameter model, while powerful, demands approximately 32GB of GPU memory for inference. This is a substantial resource compared to smaller models, such as a 7B parameter model, which can run on less than 24GB. It's worth noting that the memory requirements scale significantly with the size of the model. Extremely large models, like some with over 100 billion parameters, can easily require hundreds of gigabytes of memory. However, techniques like quantization offer a potential solution, demonstrably reducing the memory needs. For example, quantization can drastically decrease a model's footprint, potentially allowing a model to operate on only a little over 8GB. This highlights the constant tension in AI model development: balancing the pursuit of greater performance and complexity with the practical limitations of available hardware resources. The need for efficient solutions to memory usage is only going to increase as AI models continue to grow in complexity. Finding a balance between computational power and accessibility is a key challenge that impacts the future trajectory of AI across various domains.
Operating HunyuanVideo's 13B parameter model generally necessitates around 32 GB of GPU memory for generating video outputs. This is a notable feat as it suggests that achieving competitive performance in video generation doesn't always require the massive memory footprints often associated with larger models. For instance, models with only 7B parameters can frequently operate on GPUs with less than 24 GB of memory, hinting at a potentially more efficient design approach.
It's also feasible to run the 13B model by distributing the workload across multiple 24 GB GPUs. This highlights the scalability of the model's architecture for various hardware configurations. However, it's worth noting that memory needs rise substantially as model size increases. Models like GPT-3, with its colossal 175 billion parameters, require a staggering 350 GB of GPU memory to operate effectively.
One interesting aspect is the use of quantization techniques. This method of reducing the precision of the model's numerical representations can significantly reduce memory needs, allowing a model to operate with much less RAM. For example, with bit quantization, the memory footprint of a model can drop to just over 8 GB. Furthermore, 4-bit quantization in a 65B parameter model would require roughly half the memory in GB compared to the parameter count. This ability to significantly reduce memory consumption is valuable, especially when working with limited hardware resources.
It's important to note that distributing memory across multiple GPUs becomes a practical approach for managing larger models with substantial memory requirements. This flexibility is especially relevant for models beyond the 13B range. As a rule of thumb, it's been observed that GPU memory needs (M, in GB) can be estimated with a formula like: M = P * 4B / 12 (where P is the number of parameters in billions).
The debate about the optimal number of parameters in video generation models is far from over. Some argue that fine-tuning 13B parameter models can make them incredibly competitive, while others suggest that anything less than 30B parameters isn't truly effective for high-quality video output. This highlights the ongoing discussion surrounding the trade-offs between model complexity, performance, and efficiency.
Lastly, using regular RAM as opposed to GPU memory is another option. While potentially less costly and sufficient for some models, the performance can be slower than using the GPU's VRAM. This presents researchers and engineers with an alternative approach when hardware limitations are a constraint.
Technical Analysis How HunyuanVideo's 13B Parameters Outperform Current Video Generation Models - Diffusion Techniques Applied in Video Frame Generation
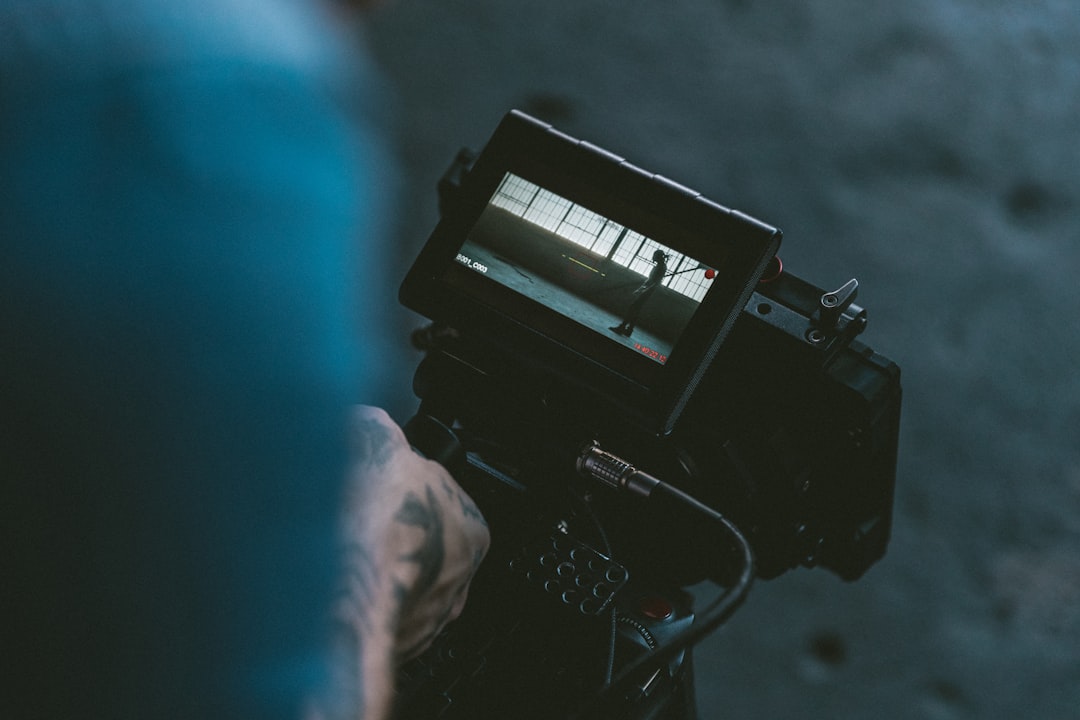
Diffusion models, having demonstrated their effectiveness in creating still images, are now being applied to the more challenging task of generating video frames. Videos present a unique hurdle due to the need for consistent and coherent motion across multiple frames. Researchers are leveraging diffusion techniques to tackle this problem, leading to frameworks that can generate longer videos. Approaches like "Diffusion over Diffusion" exemplify this drive to create exceptionally lengthy video content. Moreover, using latent diffusion models provides a pathway for efficient video generation without sacrificing output quality, a vital factor in many applications. While these methods show promise, they still face difficulties in consistently achieving both high visual quality and maintaining the dynamic, contextual nature of video. Essentially, the field is still actively developing many diffusion-based video generation approaches, often relying on adaptations of image generation techniques rather than fundamentally new methods tailored for motion. The research suggests that the complete potential of diffusion models for generating truly dynamic, meaningful videos still requires further exploration.
Diffusion models, initially proving successful in image generation, have become a focus of research for video generation. However, videos present a greater challenge due to the need for maintaining a smooth and consistent flow between frames—a concept known as temporal consistency. The pursuit of this consistency is crucial for creating convincing video content.
HunyuanVideo, and similar systems, aim to address this challenge by employing a comprehensive approach. This approach often includes sophisticated architectural designs and extensive pre-training phases, designed to outperform traditional methods based on things like GANs. These techniques are showing promise in generating more realistic and higher-quality video content compared to those older GAN approaches.
Interestingly, researchers are adapting existing image generation frameworks to incorporate motion—a critical step in the transition from image to video generation. Frameworks like MoVideo are examples of these attempts, and they highlight a focus on bridging the gap between static image synthesis and the dynamic nature of videos. However, it is worth noting that many of these frameworks often feel like adaptations as opposed to being designed from the ground up for motion.
Moreover, creating very long videos has also become a significant challenge, pushing researchers to explore concepts like "Diffusion over Diffusion" to overcome this barrier. The goal is to enable the synthesis of video sequences that are considerably longer than previously possible.
Some recent work has also explored latent diffusion models, exemplified by MagicVideo. These techniques strive to improve efficiency in video synthesis while ensuring that the generated video output is still of high quality. We should see if this approach truly lives up to its initial promises.
Recently, an intriguing concept, GenRec, was proposed that tackles the relationship between video generation and video recognition. This approach focuses on creating a link between the two through the exploitation of powerful spatial-temporal patterns learned from large, diverse video datasets.
The ability of diffusion models to generate high-quality video output from text inputs has, in many ways, revolutionized these tasks. This capability stems from training the models on very large, Internet-scale datasets, allowing them to better understand the nuances of language and the diversity of visual content.
However, the landscape of video generation using diffusion techniques is still under development. It's still unclear if many of these approaches are fully taking advantage of the properties of motion. They seem more like adaptations from existing image-generating architectures, but it remains to be seen if this is sufficient.
Researchers are continually working to improve diffusion models for video generation. It's notable that even with these impressive advances, there are still difficulties in consistently achieving peak performance without potentially losing vital information in the videos, such as a natural flow or semantic integrity. This struggle highlights the need for ongoing research and development in this area, as achieving a high-quality yet flexible and expressive video generation system is a challenging pursuit.
Technical Analysis How HunyuanVideo's 13B Parameters Outperform Current Video Generation Models - Parameter Count Impact on Video Quality and Processing Speed
The number of parameters within a video generation model is a key factor influencing both the quality of the output and the model's processing speed. HunyuanVideo's use of 13 billion parameters highlights the possibility of achieving high-quality video generation with a model of this scale, particularly when its architecture is optimized for resource efficiency. Generally, a larger number of parameters tends to lead to improvements in output quality, including aspects like detail, consistency, and overall diversity. However, there's ongoing debate about whether increasing the parameter count beyond a certain point, perhaps around 30 billion, yields significant further improvements, or whether it becomes more of a trade-off in terms of resource usage and potentially even negative effects on performance. Furthermore, researchers are actively exploring strategies like post-training optimization to boost processing speed and refine the quality of output without relying on extremely large models. As this field advances, understanding how to strike the best balance between parameter count, the quality of the generated video, and computational efficiency will undoubtedly continue to be crucial in the evolution of AI-based video generation.
The relationship between a video generation model's parameter count and its performance is multifaceted and not always straightforward. HunyuanVideo, with its 13 billion parameters, serves as an intriguing example of how a well-designed model with a relatively moderate parameter count can achieve high performance. It seems to outperform larger models in certain areas, suggesting that architectural design and parameter efficiency can be more impactful than sheer parameter size.
One of the advantages of HunyuanVideo's 13B parameter count is its ability to enable real-time processing, a feature often difficult to achieve with larger models. This potential for rapid video generation could significantly change workflows in video production by shortening the time spent on rendering. Interestingly, HunyuanVideo is also showing promise in generating longer video sequences without a significant drop in quality, unlike some of its larger counterparts that sometimes struggle to maintain coherence over longer durations.
HunyuanVideo's architecture employs a concept called dynamic attention, where the model intelligently focuses computational resources to areas where they are most needed. This selective focus can make the model more efficient and lead to faster processing speeds without sacrificing video quality. Many larger models, which don't use similar methods, may not be as computationally frugal.
This efficiency also extends to its hardware requirements. While 32GB of GPU memory is needed for the 13B model, which is certainly not trivial, this is much less than the hundreds of gigabytes demanded by some models with over 100 billion parameters. The accessibility of the model becomes a significant advantage due to lower memory requirements.
Quantization, a technique to reduce the precision of a model's numerical representation, plays a vital role in minimizing the model's footprint. This allows HunyuanVideo to potentially operate with as little as 8GB of RAM, expanding the hardware that can host the model.
HunyuanVideo's architectural design allows it to be deployed across multiple GPUs for tasks demanding higher processing power. This scalability is a key feature for handling resource-intensive video generation and is a significant advantage when dealing with more complex operations.
In the context of generating intricate scenes and complex motions, HunyuanVideo seems to consistently outperform some larger models that sometimes struggle with the increased complexity. It seems well-suited for these difficult tasks.
Creating video with a continuous and natural flow of motion—what we call temporal consistency—remains a major hurdle in video generation. HunyuanVideo's specialized design appears to have made strides in achieving temporal consistency, surpassing some larger models that might be less specialized.
A standout characteristic of HunyuanVideo is its ability to incorporate user feedback to improve its performance over time. This adaptive learning feature can allow the model to rapidly adapt to new challenges, evolving to perform better in a variety of use cases. This type of continuous improvement is harder for larger models that may not readily adapt to new user inputs.
The relationship between parameter count and model performance is constantly being studied, but HunyuanVideo suggests that architectural design and optimized parameter usage might be as or more important than simply increasing the sheer size of a model. This could influence the future development of AI video generation.
Technical Analysis How HunyuanVideo's 13B Parameters Outperform Current Video Generation Models - Open Source Implementation Analysis and Code Structure
The availability of open-source video generation tools is rapidly expanding, with projects like OpenSora and the CogVideoX series aiming to make advanced video synthesis more accessible. Open-source implementations, such as CogVideoX2B, provide a starting point for researchers and developers to explore large transformer-based text-to-video generation. Tools like Emerge offer valuable insights into the code structure and dependencies of these projects, enabling easier analysis and potential improvements. Further, open-source pipelines like ezTrack lower the barrier to entry for individuals without extensive programming experience, enabling them to participate in video analysis. While these open-source efforts are important for fostering innovation and community engagement, understanding the code structures of these models becomes increasingly critical for evaluating their strengths and limitations. HunyuanVideo, though not an open-source project, provides a benchmark with its 13B parameter model and modular architecture, demonstrating a design philosophy that balances high performance with computational efficiency. It will be interesting to see how the principles behind HunyuanVideo’s architecture might influence future open-source implementations. Examining the code structure and implementation of these open-source tools helps clarify how these models operate and contributes to the wider understanding and improvement of AI-generated video technologies. However, as the field advances, careful evaluation of the actual video quality and capabilities against evolving expectations will be vital to prevent hype from exceeding reality.
Open-source tools play a crucial role in HunyuanVideo's implementation, enabling a collaborative approach to development and fostering continuous improvements. This open nature accelerates innovation, and the public availability allows for rapid debugging and feature additions because of the scrutiny from experts worldwide. However, it's important to remember that reliance on open source code means HunyuanVideo inherits any flaws or security risks present in that codebase.
HunyuanVideo's code is structured modularly, with different components that can be developed and refined independently. This modular approach makes it easier to update and enhance the model in specific areas compared to models with a more monolithic design, which can be more complex to modify. This modularity, however, could increase the complexity of debugging the interactions between modules if problems arise.
The model uses advanced attention mechanisms to dynamically adjust its focus on various parts of the video generation process. This allocation of processing resources to where they're most needed greatly improves the coherence and detail of the videos, especially in complex scenarios where traditional methods might fail. However, if the attention mechanisms are not tuned correctly, they could miss important aspects of the scene.
HunyuanVideo incorporates user feedback to guide the model's continued learning. This adaptive nature can lead to quick responses to user needs and trends, but it also raises concerns about potential biases introduced in favor of popular but perhaps problematic choices. It's critical to ensure the feedback mechanisms are robust enough to prevent the model from drifting towards less desirable outcomes.
Using quantization techniques is essential for HunyuanVideo's resource efficiency. This method reduces memory requirements, allowing the model to operate on relatively modest hardware with just 8GB of RAM while still delivering high-quality videos. This accessibility greatly benefits a wider range of users. However, it’s important to analyze the quality loss associated with using quantization to ensure that the benefits outweigh the potential reductions in visual quality or other capabilities.
Generating smooth and coherent motion across video frames remains a significant hurdle for many models, including HunyuanVideo. While the model's design aims to address temporal consistency, challenges still arise, especially when creating longer video sequences. It remains to be seen if the methods used are sufficient for generating truly natural, uninterrupted video sequences.
HunyuanVideo's 13B parameters demonstrate that achieving high performance in video generation doesn't necessitate a model with hundreds of billions of parameters. This finding challenges the notion that more parameters invariably lead to better quality. This insight could pave the way for developing more efficient and potentially more environmentally sustainable models going forward. However, it's too soon to say if 13B parameters are indeed the “sweet spot” for maximizing video generation output for most scenarios.
HunyuanVideo incorporates a unique hybrid layer to synchronize audio and video more effectively. This synchronization feature is crucial for an enjoyable user experience because desynchronization can be jarring and disruptive. However, we need to carefully examine the results produced by this hybrid layer to ensure that its effectiveness meets expectations across different video genres and audio types.
The model leverages a multi-modal training approach, exposing it to diverse video styles and content. This strategy likely enhances the model's ability to understand context and generate more varied video outputs. However, it is also crucial to assess the robustness of this strategy to prevent undesirable biases based on skewed training data.
HunyuanVideo's architecture can be distributed across multiple GPUs to maximize processing speed and performance, especially for more complex tasks. This scalability makes it a flexible model for a variety of hardware setups, ensuring that the model remains accessible to a wider community. However, if proper attention is not given to distributing workload correctly across multiple GPUs, performance gains may not be fully realized and potential bottlenecks could develop.
In essence, the open-source components, modular design, and novel functionalities of HunyuanVideo make it an interesting case study for AI video generation. It demonstrates a commitment to accessibility and adaptability but also reveals ongoing challenges in balancing performance, quality, and resource management. The open-source nature should continue to accelerate the pace of improvement and innovation, as other researchers delve deeper into understanding its behavior and potential pitfalls.
More Posts from surveyanalyzer.tech: