Unlock the power of survey data with AI-driven analysis and actionable insights. Transform your research with surveyanalyzer.tech. (Get started for free)
Machine Learning Career Outlook 2025 Entry Points and Salary Realities Beyond the Hype
Machine Learning Career Outlook 2025 Entry Points and Salary Realities Beyond the Hype - Entry Level Machine Learning Engineer Salaries Range From 115k to 135k in 2024
Beginning machine learning engineers in the US can currently expect to earn between $115,000 and $135,000 annually. This salary range signifies a growing need for individuals with these skills. While the average reported salary hovers around $127,350, it's crucial to realize that compensation can vary significantly. The geographical location of a role plays a large part, with some areas offering much higher pay. Consequently, those seeking these roles need to be thoughtful about where they apply to get the best possible salary. Even with the positive outlook for entry-level machine learning jobs, it's a wise move for aspiring engineers to think critically about their career choices and prioritize maximizing their earnings where possible.
Based on current data, it seems that a starting point for machine learning engineers in 2024 falls within a range of $115,000 to $135,000. This figure, while seemingly attractive, can vary depending on several factors. It's interesting to note that this range is a snapshot in time and can fluctuate as the field evolves. The data indicates a wide spread in salaries across the industry, potentially highlighting the impact of experience level, location, and specific industry on what a company is willing to offer. It's not surprising that companies are competing to attract talent, which may lead to a more generous entry-level salary in certain circumstances. However, we must also consider that this salary range could be skewed by factors such as the inclusion of stock options or bonuses, which can inflate the overall compensation package. It would be helpful to have more granular data that separates base salary from these other components to get a more accurate understanding of what to realistically anticipate. One could argue that this range, especially the higher end, may not be representative of the average starting salary. Further analysis is likely necessary to understand the distribution within this range and whether it truly reflects the salary experiences of a majority of entry-level machine learning engineers. The market seems to be dynamic, with a combination of demand and talent scarcity shaping the landscape. While it appears promising on the surface, it's wise to be aware of the potential variations and consider the broader picture of the compensation package when assessing the attractiveness of a particular opportunity.
Machine Learning Career Outlook 2025 Entry Points and Salary Realities Beyond the Hype - Computer Vision Specialist Role Grows 78 Percent Since 2023
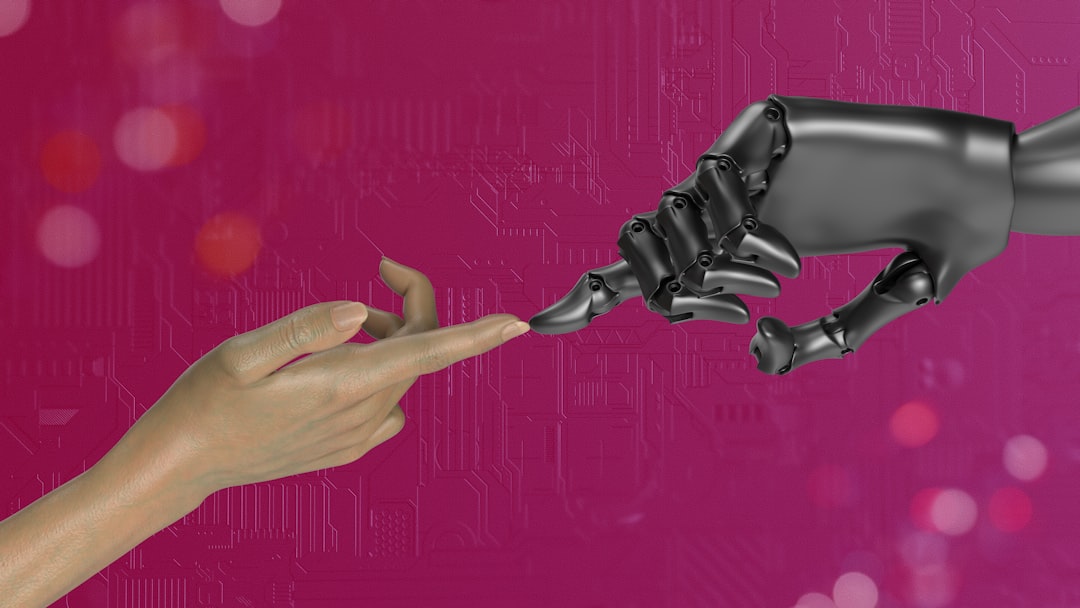
The demand for Computer Vision Specialists has surged, with a remarkable 78% increase in roles since 2023. This growth is mirrored in the broader computer vision market, which is expected to nearly quadruple in size by 2026. While these trends paint a picture of opportunity, it's worth noting that entry-level salaries for computer vision engineers, around $65,231, might not be as high as those in other machine learning areas. The field is experiencing rapid evolution, driven by developments like real-time processing and adaptable learning systems. How these advancements reshape the job market for computer vision specialists remains to be seen. Ultimately, the increasing use of computer vision across various industries, from security to healthcare, suggests that collaboration and innovation will be vital for those hoping to leverage its capabilities. It will be interesting to observe how this field develops and whether its salary trajectory aligns with its significant growth.
The field of computer vision has seen a remarkable surge in demand, with the number of specialist roles expanding by a significant 78% since 2023. This growth is fueled by continuous advancements in image processing techniques and the increasing integration of computer vision into diverse sectors like healthcare, transportation, and security.
For example, self-driving car systems are incorporating computer vision at an accelerating pace. It's predicted that these systems could decrease traffic accidents by more than 30% in the coming years due to enhanced perception and environmental awareness. These developments, coupled with projections placing the global market value for computer vision at over $19 billion by 2025, suggest a promising outlook for career opportunities in this area.
It's interesting to note that the growth in demand isn't limited to just tech companies. Retail businesses are increasingly adopting computer vision for streamlining operations like inventory management and enabling checkout-free shopping. This broad adoption underscores the versatility of the technology and its potential across a wide array of industries.
The core of many computer vision applications is deep learning, specifically models that rely on convolutional neural networks (CNNs). Research indicates that these models are achieving high levels of accuracy – upwards of 90% – in complex image classification tasks. This reliance on deep learning implies a need for specialists who possess a strong understanding of these models and their application to real-world problems.
Consequently, collaboration between different disciplines is becoming increasingly important. Computer vision engineers are frequently working alongside data scientists and software engineers to develop integrated solutions that push the boundaries of traditional approaches. This collaborative approach is shaping a new type of job landscape in which specialists need to be comfortable with cross-functional teamwork.
Further, as AI-powered devices become more prevalent in everyday life, a foundational understanding of computer vision is becoming vital not only for dedicated specialists but also for a broader range of engineers entering the field. Despite this expanding need, a significant skills gap exists, with many employers reporting difficulty finding candidates with the required computer vision and machine learning expertise.
While strong technical foundations in programming and algorithm development are essential, soft skills are also rising in importance. Communication and collaboration are becoming crucial as computer vision engineers increasingly find themselves working within multidisciplinary teams.
Finally, it's worth observing that the geographic location plays a key role in the salary landscape. Cities with established tech hubs, such as San Francisco and Seattle, currently offer salaries for computer vision specialists significantly above the national average. This disparity is likely a reflection of factors such as the higher cost of living and highly competitive job markets in these regions.
Machine Learning Career Outlook 2025 Entry Points and Salary Realities Beyond the Hype - Natural Language Processing Skills Drive 45k Salary Premium
Individuals with expertise in Natural Language Processing (NLP) are seeing a significant financial advantage in the job market, with salaries averaging around $187,143. This represents a roughly $45,000 bump compared to the average machine learning engineer. The growing need for individuals skilled in NLP reflects a broader shift in AI and machine learning, where these specialized abilities are becoming increasingly valuable to employers. While the financial incentives are attractive, it's important to recognize that developing a strong foundation in NLP requires a deep understanding of linguistics – concepts like syntax and semantics are key – coupled with strong programming skills, especially in Python. The trajectory for NLP specialists seems promising, suggesting a strong link between this specialized expertise and career success, both in terms of higher earning potential and increased job opportunities across a wide range of industries. However, it's crucial to understand that this high-paying niche is competitive, and individuals must continually update their skills as the field progresses.
The ability to work with natural language processing (NLP) seems to be a valuable asset in the current job market, as it's tied to a substantial salary increase. It appears that individuals with NLP skills can command an average of roughly $187,143 per year, which is about $45,000 more than the average machine learning engineer salary. This salary difference suggests that there's a shortage of people who can effectively use NLP in real-world applications. Businesses seem to be recognizing that NLP helps them make better decisions with data, and that can translate to greater productivity, for example, improvements in customer interactions and other vital metrics.
The recent surge in investments in areas like deep learning and transformer models has accelerated the progress of NLP. This advancement has improved the quality of tasks like sentiment analysis and machine translation, leading to higher salaries for people with these specific skill sets due to their expanding uses in many different fields. While large tech companies have traditionally been at the forefront of NLP, industries like finance and healthcare are starting to see the advantages it offers. This trend is leading to more competitive pay for NLP professionals outside the typical tech companies.
Reports forecast that the demand for NLP experts will grow by around 25% until 2025, leading to an expanding job market and higher compensation for those who can use NLP technology effectively. It's intriguing how the field is becoming more specialized, with subfields like chatbot and speech recognition each having distinct salary levels, depending on the complexity of the tasks and where they are being applied.
Combining NLP knowledge with expertise in other fields, like data science or software development, seems to increase an individual's negotiating power, as these complementary abilities are highly valued. This observation highlights the importance of combining skills in a tight job market. However, there is also a noticeable skills gap. Many companies have difficulty filling NLP roles because they struggle to find individuals who have both the technical know-how and practical experience required for advanced NLP projects.
The location of a role appears to play a part in the salary situation, too. We see a difference in salary levels between metropolitan areas and rural locations, with tech-heavy urban centers tending to offer more generous compensation. This disparity seems to reflect the concentration of tech talent and resources in these areas. While the information paints a picture of exciting opportunities for people interested in NLP, it's important to look beyond just the average salary and acknowledge these factors that can influence individual compensation.
Machine Learning Career Outlook 2025 Entry Points and Salary Realities Beyond the Hype - Remote Machine Learning Jobs Make Up 67 Percent of New Positions
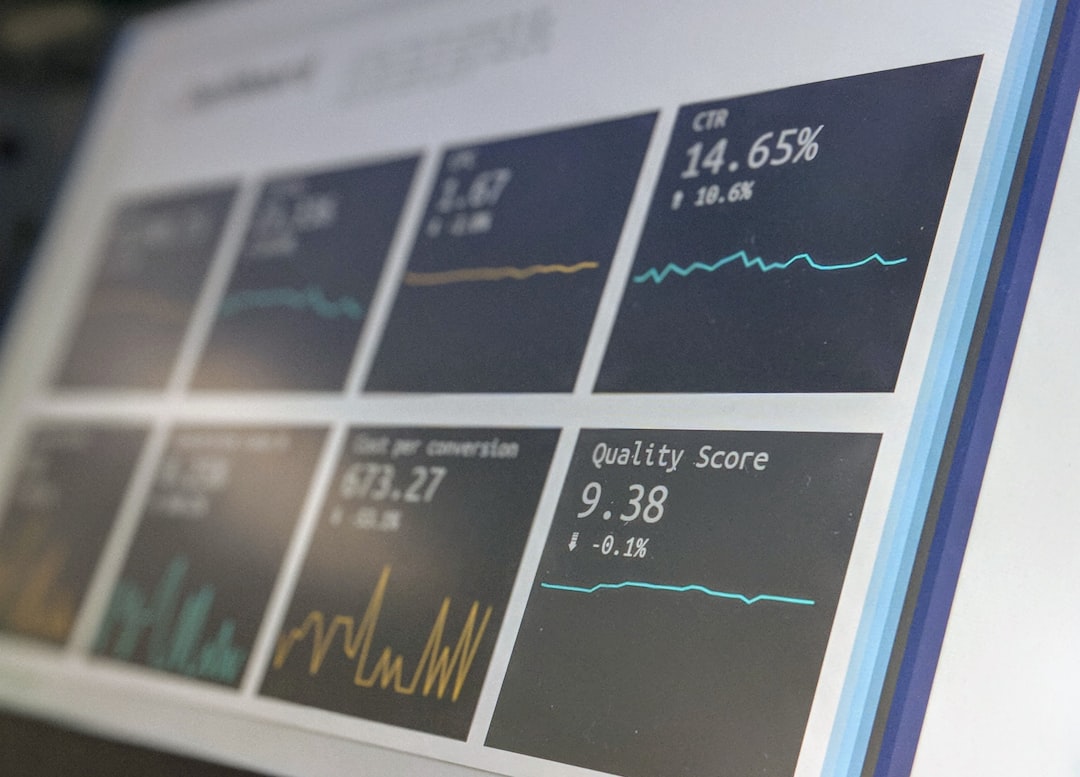
The field of machine learning is experiencing a significant shift towards remote work, with a substantial 67% of new positions being remote. This trend reflects a growing preference among companies to hire remotely, likely fueled by technological advancements and the competitive nature of the talent pool. Remote machine learning engineer roles are also financially attractive, with average salaries reported around $171,876 annually. The sheer number of available remote machine learning jobs, estimated at over 28,000, underscores the robust demand for skilled individuals in this space. Looking ahead to 2025, the outlook for machine learning careers remains positive, showcasing growth and a range of entry points for individuals seeking to enter the field. This dynamic and evolving landscape highlights how remote work is a fundamental aspect of the future of machine learning careers. While promising, it's important to acknowledge that salary can vary greatly depending on experience, location and specific industry, making careful consideration of job opportunities crucial for maximizing earnings.
The landscape of machine learning jobs is experiencing a notable shift towards remote work, with a striking 67% of new positions now being offered remotely. This trend, driven by factors like evolving workplace preferences and advancements in technology that facilitate distributed collaboration, is reshaping the way machine learning projects are conceived and executed. It's no longer just a phenomenon confined to tech companies either; traditional industries like finance and healthcare are increasingly incorporating remote machine learning expertise to bolster their operations and drive innovation.
While the allure of remote work is undeniable, especially for those seeking flexibility and a better work-life balance, it also introduces complexities surrounding team collaboration. Some engineers believe that in-person interactions can cultivate a unique level of creativity and idea exchange that's often difficult to replicate in a virtual environment. Furthermore, the absence of direct supervision inherent in remote work often demands heightened self-management skills. Individuals in these positions must possess exceptional time management and project prioritization abilities, potentially leading to different performance benchmarks compared to in-office roles.
It's notable that the appeal of remote machine learning work spans various demographic groups. A considerable portion of young engineers are actively seeking out remote roles as part of their career plans, reflecting a change in the expectations of today's tech workforce. The advantages of expanding the talent pool beyond geographical boundaries are becoming increasingly evident to employers. Companies can access a wider spectrum of skills and perspectives from a more diverse range of individuals, a clear benefit in a competitive talent market.
The compensation structure for remote machine learning roles, however, is not uniform. While some companies offer competitive salaries comparable to their on-site positions, others still maintain a location-based pay structure. This practice can inadvertently create disparities and potentially disadvantage remote workers, an issue that's been a subject of growing concern. The increasing number of remote positions has also led to a heightened emphasis on asynchronous communication abilities in job descriptions. Organizations are seeking engineers adept at articulating their ideas and findings through written communications, a skill that's essential for maintaining clear and efficient workflows in a geographically dispersed team.
The emergence of remote work in machine learning has inevitably sparked discussions on the future of team dynamics and collaborative workflows. While the flexibility offered by remote roles is undeniable, concerns linger regarding team cohesion and the effectiveness of knowledge sharing across geographically distant groups. It's a challenge that the field is beginning to grapple with as the trend towards remote work gains momentum. Balancing these factors while maintaining both innovation and effective team functioning will be a key issue for organizations and individuals alike in the evolving landscape of machine learning.
Machine Learning Career Outlook 2025 Entry Points and Salary Realities Beyond the Hype - Alternative Career Paths Through MLOps and ML Infrastructure Jobs
Within the broader machine learning landscape, pursuing a career path focused on MLOps and machine learning infrastructure provides an interesting alternative. The field of MLOps, which encompasses the practices and processes involved in deploying, maintaining, and monitoring machine learning models in production, is seeing significant growth. Predictions indicate the MLOps market will expand substantially, from $38 billion in 2021 to a projected $211 billion by 2026, indicating a rising demand for individuals with these skills. These roles demand expertise in managing the entire machine learning lifecycle, requiring collaboration between different types of specialists, including data scientists, software engineers, and operations professionals. It's a space that emphasizes bridging the gap between machine learning development and its practical deployment, making it essential for translating AI projects from theory to real-world execution.
While MLOps jobs often provide compensation on par with traditional machine learning roles, it's important to recognize the need to adapt and continually learn in this quickly evolving domain. The technical complexities of maintaining and scaling machine learning systems pose significant challenges, demanding a combination of deep technical understanding, operational fluency, and a willingness to adapt to the latest developments in the field. Those comfortable navigating these challenges, and who can effectively bridge the domains of machine learning and production, can carve out meaningful and potentially lucrative career paths in this space.
The field of machine learning is evolving rapidly, with MLOps and ML infrastructure roles emerging as significant career paths. Individuals with backgrounds in cloud computing and DevOps often find their skills are readily applicable in these roles, leading to a smoother learning curve and potentially higher starting salaries. This is because they bring a unique perspective that blends traditional software engineering with machine learning expertise.
It's notable that project management capabilities are becoming increasingly important in MLOps positions, especially when considering the intricate process of deploying, monitoring, and scaling machine learning models. As a result, companies are sometimes looking beyond the standard data science pool to find candidates with strong organizational and coordination skills, which is an interesting development in the field.
The increasing importance of MLOps has led to a proliferation of tools and frameworks, which are largely cloud-based and constantly changing. Developing a working knowledge of these platforms, such as Kubeflow and MLflow, is becoming crucial for job seekers, as companies prioritize candidates who are comfortable with the most relevant tools.
However, the field is not purely technical. Soft skills like communication and collaboration are becoming highly valued. The complexity of many MLOps tasks often necessitates strong communication skills, especially in cross-functional teams. Employers are recognizing that the ability to clearly explain technical concepts is critical for fostering productive interactions and innovation.
The financial incentives for entry-level MLOps roles are competitive, particularly in prominent tech areas, with potential starting salaries reaching $130,000 or more. However, these positions demand a more holistic understanding than just technical proficiency. The best candidates demonstrate a deep grasp of both operational processes and the overall context within an organization.
The demand for individuals with MLOps expertise is projected to increase by about 32% through 2025. This rise is reflective of the wider industry's increasing realization that deploying and maintaining machine learning models effectively requires well-structured processes.
In a further intriguing development, many companies are starting to acknowledge the significance of ethical considerations in AI deployments. Consequently, there's a growing need for MLOps engineers to contribute to the creation of responsible governance frameworks. This could present unexpected opportunities for those with backgrounds in ethics or compliance.
Implementing continuous integration and continuous deployment (CI/CD) pipelines in the context of machine learning often presents a significant hurdle for teams. MLOps engineers often have to develop innovative solutions to automate these processes, potentially playing a critical role in streamlining traditionally cumbersome workflows.
As machine learning integrates further into business operations, leadership is looking for MLOps professionals who can bridge the gap between technological capabilities and strategic business objectives. This evolving landscape is paving the way for those who have both strong technical backgrounds and a grasp of business principles to move quickly through the organizational hierarchy.
Finally, the widespread adoption of MLOps principles is causing a subtle but notable shift in job descriptions across a range of industries. We are starting to see traditional data scientists assuming more operational responsibilities, while software engineers are increasingly faced with complex machine learning algorithms. This is leading to a more blended approach to job roles, a situation that wasn't as common only a few years ago. It will be interesting to see how these evolving roles continue to shape the future of the field.
Unlock the power of survey data with AI-driven analysis and actionable insights. Transform your research with surveyanalyzer.tech. (Get started for free)
More Posts from surveyanalyzer.tech: